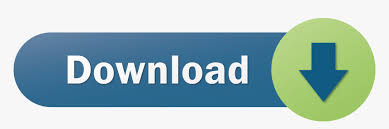
Create deepfake videos Archives
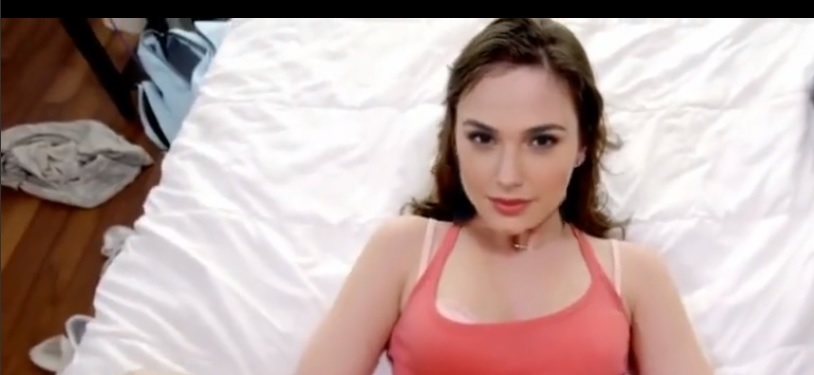
create deepfake videos Archives
Internet Companies Prepare to Fight the ‘Deepfake’ Future
Researchers are creating tools to find A.I.-generated fake videos before they become impossible to detect. Some experts fear it is a losing battle.
SAN FRANCISCO — Several months ago, Google hired dozens of actors to sit at a table, stand in a hallway and walk down a street while talking into a video camera.
Then the company’s researchers, using a new kind of artificial intelligence software, swapped the faces of the actors. People who had been walking were suddenly at a table. The actors who had been in a hallway looked like they were on a street. Men’s faces were put on women’s bodies. Women’s faces were put on men’s bodies. In time, the researchers had created hundreds of so-called deepfake videos.
By creating these digitally manipulated videos, Google’s scientists believe they are learning how to spot deepfakes, which researchers and lawmakers worry could become a new, insidious method for spreading disinformation in the lead-up to the 2020 presidential election.
For internet companies like Google, finding the tools to spot deepfakes has gained urgency. If someone wants to spread a fake video far and wide, Google’s YouTube or Facebook’s social media platforms would be great places to do it.
Imagine a fake Senator Elizabeth Warren, virtually indistinguishable from the real thing, getting into a fistfight in a doctored video. Or a fake President Trump doing the same. The technology capable of that trickery is edging closer to reality.
“Even with current technology, it is hard for some people to tell what is real and what is not,” said Subbarao Kambhampati, a professor of computer science at Arizona State University.
On ‘The Weekly,’ A.I. Engineers Create a Deepfake Video
[Sunday at 10 p.m. on FX and Streaming Monday on Hulu.]
Deepfakes — a term that generally describes videos doctored with cutting-edge artificial intelligence — have already challenged our assumptions about what is real and what is not.
In recent months, video evidence was at the center of prominent incidents in Brazil, Gabon in Central Africa and China. Each was colored by the same question: Is the video real? The Gabonese president, for example, was out of the country for medical care and his government released a so-called proof-of-life video. Opponents claimed it had been faked. Experts call that confusion “the liar’s dividend.”
“You can already see a material effect that deepfakes have had,” said Nick Dufour, one of the Google engineers overseeing the company’s deepfake research. “They have allowed people to claim that video evidence that would otherwise be very convincing is a fake.”
For decades, computer software has allowed people to manipulate photos and videos or create fake images from scratch. But it has been a slow, painstaking process usually reserved for experts trained in the vagaries of software like Adobe Photoshop or After Effects.
Now, artificial intelligence technologies are streamlining the process, reducing the cost, time and skill needed to doctor digital images. These A.I. systems learn on their own how to build fake images by analyzing thousands of real images. That means they can handle a portion of the workload that once fell to trained technicians. And that means people can create far more fake stuff than they used to.
The technologies used to create deepfakes is still fairly new and the results are often easy to notice. But the technology is evolving. While the tools used to detect these bogus videos are also evolving, some researchers worry that they won’t be able to keep pace.
Google recently said that any academic or corporate researcher could download its collection of synthetic videos and use them to build tools for identifying deepfakes. The video collection is essentially a syllabus of digital trickery for computers. By analyzing all of those images, A.I. systems learn how to watch for fakes. Facebook recently did something similar, using actors to build fake videos and then releasing them to outside researchers.
Engineers at a Canadian company called Dessa, which specializes in artificial intelligence, recently tested a deepfake detector that was built using Google’s synthetic videos. It could identify the Google videos with almost perfect accuracy. But when they tested their detector on deepfake videos plucked from across the internet, it failed more than 40 percent of the time.
They eventually fixed the problem, but only after rebuilding their detector with help from videos found “in the wild,” not created with paid actors — proving that a detector is only as good as the data used to train it.
Their tests showed that the fight against deepfakes and other forms of online disinformation will require nearly constant reinvention. Several hundred synthetic videos are not enough to solve the problem, because they don’t necessarily share the characteristics of fake videos being distributed today, much less in the years to come.
“Unlike other problems, this one is constantly changing,” said Ragavan Thurairatnam, Dessa’s founder and head of machine learning.
In December 2017, someone calling themselves “deepfakes” started using A.I. technologies to graft the heads of celebrities onto nude bodies in pornographic videos. As the practice spread across services like Twitter, Reddit and PornHub, the term deepfake entered the popular lexicon. Soon, it was synonymous with any fake video posted to the internet.
The technology has improved at a rate that surprises A.I. experts, and there is little reason to believe it will slow. Deepfakes should benefit from one of the few tech industry axioms that have held up over the years: Computers always get more powerful and there is always more data. That makes the so-called machine-learning software that helps create deepfakes more effective.
“It is getting easier, and it will continue to get easier. There is no doubt about it,” said Matthias Niessner, a professor of computer science at the Technical University of Munich who is working with Google on its deepfake research. “That trend will continue for years.”
The question is: Which side will improve more quickly?
Researchers like Dr. Niessner are working to build systems that can automatically identify and remove deepfakes. This is the other side of the same coin. Like deepfake creators, deepfake detectors learn their skills by analyzing images.
Detectors can also improve by leaps and bounds. But that requires a constant stream of new data representing the latest deepfake techniques used around the internet, Dr. Niessner and other researchers said. Collecting and sharing the right data can be difficult. Relevant examples are scarce, and for privacy and copyright reasons, companies cannot always share data with outside researchers.
Though activists and artists occasionally release deepfakes as a way of showing how these videos could shift the political discourse online, these techniques are not widely used to spread disinformation. They are mostly used to spread humor or fake pornography, according to Facebook, Google and others who track the progress of deepfakes.
Right now, deepfake videos have subtle imperfections that can be readily detected by automated systems, if not by the naked eye. But some researchers argue that the improved technology will be powerful enough to create fake images without these tiny defects. Companies like Google and Facebook hope they will have reliable detectors in place before that happens.
“In the short term, detection will be reasonably effective,” said Mr. Kambhampati, the Arizona State professor. “In the longer term, I think it will be impossible to distinguish between the real pictures and the fake pictures.”
Facebook just released a database of 100,000 deepfakes to teach AI how to spot them
Social-media companies are concerned that deepfakes could soon flood their sites. But detecting them automatically is hard. To address the problem, Facebook wants to use AI to help fight back against AI-generated fakes. To train AIs to spot manipulated videos, it is releasing the largest ever data set of deepfakes—more than 100,000 clips produced using 3,426 actors and a range of existing face-swapping techniques.
“Deepfakes are currently not a big issue,” says Facebook’s CTO, Mike Schroepfer. “But the lesson I learned the hard way over last couple years is not to be caught flat-footed. I want to be really prepared for a lot of bad stuff that never happens rather than the other way around.”
Facebook has also announced the winner of its Deepfake Detection Challenge, in which 2,114 participants submitted around 35,000 models trained on its data set. The best model, developed by Selim Seferbekov, a machine-learning engineer at mapping firm Mapbox, was able to detect whether a video was a deepfake with 65% accuracy when tested on a set of 10,000 previously unseen clips, including a mix of new videos generated by Facebook and existing ones taken from the internet.
To make things harder, the training set and test set include videos that a detection system might be confused by, such as people giving makeup tutorials, and videos that have been tweaked by pasting text and shapes over the speakers’ faces, changing the resolution or orientation, and slowing them down.
Rather than learning forensic techniques, such as looking for digital fingerprints in the pixels of a video left behind by the deepfake generation process, the top five entries seem to have learned to spot when something looked “off,” as a human might do.
To do this, the winners all used a new type of convolutional neural network (CNN) developed by Google researchers last year, called EfficientNets. CNNs are commonly used to analyze images and are good at detecting faces or recognizing objects. Improving their accuracy beyond a certain point can require ad hoc fine-tuning, however. EfficientNets provide a more structured way to tune, making it easier to develop more accurate models. But exactly what it is that makes them outperform other neural networks on this task isn’t clear, says Seferbekov.
Facebook does not plan to use any of the winning models on its site. For one thing, 65% accuracy is not yet good enough to be useful. Some models achieved more than 80% accuracy with the training data, but this dropped when pitted against unseen clips. Generalizing to new videos, which can include different faces swapped in using different techniques, is the hardest part of the challenge, says Seferbekov.
He thinks that one way to improve detection would be to focus on the transitions between video frames, tracking them over time. “Even very high-quality deepfakes have some flickering between frames,” says Seferbekov. Humans are good at spotting these inconsistencies, especially in footage of faces. But catching these telltale defects automatically will require larger and more varied training data and a lot more computing power. Seferbekov tried to track these frame transitions but couldn’t. “CPU was a real bottleneck there,” he says.
Facebook suggests that deepfake detection may also be improved by using techniques that go beyond the analysis of an image or video itself, such as assessing its context or provenance.
Sam Gregory, who directs Witness, a project that supports human rights activists in their use of video technologies, welcomes the investment of social-media platforms in deepfake detection. Witness is a member of Partnership on AI, which advised Facebook on its data set. Gregory agrees with Schroepfer that it is worth preparing for the worst. “We haven’t had the deepfake apocalyps,e but these tools are a very nasty addition to gender-based violence and misinformation,” he says. For example, the DeepTrace Labs report found that 96% of deepfakes were nonconsensual pornography, in which other people’s faces are pasted over those of performers in porn clips.
When millions of people are able to create and share videos, trusting what we see is more important than ever. Fake news spreads through Facebook like wildfire, and the mere possibility of deepfakes sows doubt, making us more likely to question genuine footage as well as fake.
What’s more, automatic detection may soon be our only option. “In the future we will see deepfakes that cannot be distinguished by humans,” says Seferbekov.
What do we do about deepfake video?
There exist, on the internet, any number of videos that show people doing things they never did. Real people, real faces, close to photorealistic footage; entirely unreal events.
These videos are called deepfakes, and they’re made using a particular kind of AI. Inevitably enough, they began in porn – there is a thriving online market for celebrity faces superimposed on porn actors’ bodies – but the reason we’re talking about them now is that people are worried about their impact on our already fervid political debate. Those worries are real enough to prompt the British government and the US Congress to look at ways of regulating them.
The rise of the deepfake and the threat to democracy
The video that kicked off the sudden concern last month was, in fact, not a deepfake at all. It was a good old-fashioned doctored video of Nancy Pelosi, the speaker of the US House of Representatives. There were no fancy AIs involved; the video had simply been slowed down to about 75% of its usual speed, and the pitch of her voice raised to keep it sounding natural. It could have been done 50 years ago. But it made her look convincingly drunk or incapable, and was shared millions of times across every platform, including by Rudi Giuliani – Donald Trump’s lawyer and the former mayor of New York.
It got people worrying about fake videos in general, and deepfakes in particular. Since the Pelosi video came out, a deepfake of Mark Zuckerberg apparently talking about how he has “total control of billions of people’s stolen data” and how he “owe[s] it all to Spectre”, the product of a team of satirical artists, went viral as well. Last year, the Oscar-winning director Jordan Peele and his brother-in-law, BuzzFeed CEO Jonah Peretti, created a deepfake of Barack Obama apparently calling Trump a “complete and utter dipshit” to warn of the risks to public discourse.
A lot of our fears about technology are overstated. For instance, despite worries about screen time and social media, in general, high-quality research shows that there’s little evidence of it having a major impact on our mental health. Every generation has its techno-panic: video nasties, violent computer games, pulp novels.
But, says Sandra Wachter, a professor in the law and ethics of AI at the Oxford Internet Institute, deepfakes might be a different matter. “I can understand the public concern,” she says. “Any tech developing so quickly could have unforeseen and unintended consequences.” It’s not that fake videos or misinformation are new, but things are changing so fast, she says, that it’s challenging our ability to keep up. “The sophisticated way in which fake information can be created, how fast it can be created, and how endlessly it can be disseminated is on a different level. In the past, I could have spread lies, but my range was limited.”
Here’s how deepfakes work. They are the product of not one but two AI algorithms, which work together in something called a “generative adversarial network”, or Gan. The two algorithms are called the generator and the discriminator.
Imagine a Gan that has been designed to create believable spam emails. The discriminator would be exactly the same as a real spam filter algorithm: it would simply sort all emails into either “spam” or “not spam”. It would do that by being given a huge folder of emails, and determining which elements were most often associated with the ones it was told were spam: perhaps words like “enlarger” or “pills” or “an accident that wasn’t your fault”. That folder is its “training set”. Then, as new emails came in, it would give each one a rating based on how many of these features it detected: 60% likely to be spam, 90% likely, and so on. All emails above a certain threshold would go into the spam folder. The bigger its training set, the better it gets at establishing real from fake.
But the generator algorithm works the other way. It takes that same dataset and uses it to build new emails that don’t look like spam. It knows to avoid words like “penis” or “won an iPad”. And when it makes them, it puts them into the stream of data going through the discriminator. The two are in competition: if the discriminator is fooled, the generator “wins”; if it isn’t, the discriminator “wins”. And either way, it’s a new piece of data for the Gan. The discriminator gets better at telling fake from real, so the generator has to get better at creating the fakes. It is an arms race, a self-reinforcing cycle. This same system can be used for creating almost any digital product: spam emails, art, music – or, of course, videos.
Gans are hugely powerful, says Christina Hitrova, a researcher in digital ethics at the Alan Turing Institute for AI, and have many interesting uses – they’re not just for creating deepfakes. The photorealistic imaginary people at ThisPersonDoesNotExist.com are all created with Gans. Discriminatory algorithms (such as spam filters) can be improved by Gans creating ever better things to test them with. It can do amazing things with pictures, including sharpening up fuzzy ones or colourising black-and-white ones. “Scientists are also exploring using Gans to create virtual chemical molecules,” says Hitrova, “to speed up materials science and medical discoveries: you can generate new molecules and simulate them to see what they can do.” Gans were only invented in 2014, but have already become one of the most exciting tools in AI.
But they are widely available, easy to use, and increasingly sophisticated, able to create ever more believable videos. “There’s some way to go before the fakes are undetectable,” says Hitrova. “For instance, with CGI faces, they haven’t quite perfected the generation of teeth or eyes that look natural. But this is changing, and I think it’s important that we explore solutions – technological solutions, and digital literacy solutions, as well as policy solutions.”
With Gans, one technological solution presents itself immediately: simply use the discriminator to tell whether a given video is fake. But, says Hitrova, “Obviously that’s going to feed into the fake generator to produce even better fakes.” For instance, she says, one tool was able to identify deepfakes by looking at the pattern of blinking. But then the next generation will take that into account, and future discriminators will have to use something else. The arms race that goes on inside Gans will go on outside, as well.
Other technological solutions include hashing – essentially a form of digital watermarking, giving a video file a short string of numbers which is lost if the video is tampered with – or, controversially, “authenticated alibis”, wherein public figures constantly record where they are and what they’re doing, so that if a deepfake circulates apparently showing them doing something they want to disprove, they can show what they were really doing. That idea has been tentatively floated by the AI law specialist Danielle Citron, but as Hitrova points out, it has “dystopian” implications.
None of these solutions can entirely remove the risk of deepfakes. Some form of authentification may work to tell you that certain things are real, but what if someone wants to deny the reality of something real? If there had been deepfakes in 2016, says Hitrova, “Trump could have said, ‘I never said ‘grab them by the pussy’.” Most would not have believed him – it came from Access Hollywood tapes and was confirmed by the show’s presenter – but it would have given an excuse for people to doubt them.
Education – critical thinking and digital literacy – will be important too. Finnish children score highly on their ability to spot fake news, a trait that is credited to the country’s policy of teaching critical thinking skills at school. But that can only be part of the solution. For one thing, most of us are not at school. Even if the current generation of schoolchildren becomes more wary – as they naturally are anyway, having grown up with digital technology – their elders will remain less so, as can be seen in the case of British MPs being fooled by obvious fake tweets. “Older people are much less tech-savvy,” says Hitrova. “They’re much more likely to share something without fact-checking it.”
Wachter and Hitrova agree that some sort of regulatory framework will be necessary. Both the US and the UK are grappling with the idea. At the moment, in the US, social media platforms are not held responsible for their content. Congress is considering changing that, and making such immunity dependent on “reasonable moderation practices”. Some sort of requirement to identify fake content has also been floated.
Wachter says that something like copyright, by which people have the right for their face not to be used falsely, may be useful, but that by the time you’ve taken down a deepfake, the reputational damage may already be done, so preemptive regulation is needed too.
A European Commission report two weeks ago found that digital disinformation was rife in the recent European elections, and that platforms are failing to take steps to reduce it. Facebook, for instance, has entirely washed its hands of responsibility for fact-checking, saying that it will only take down fake videos after a third-party fact-checker has declared it to be false.
Britain, though, is taking a more active role, says Hitrova. “The EU is using the threat of regulation to force platforms to self-regulate, which so far they have not,” she says. “But the UK’s recent online harms white paper and the Department for Digital, Culture, Media and Sport subcommittee [on disinformation, which has not yet reported but is expected to recommend regulation] show that the UK is really planning to regulate. It’s an important moment; they’ll be the first country in the world to do so, they’ll have a lot of work – it’s no simple task to balance fake news against the rights to parody and art and political commentary – but it’s truly important work.” Wachter agrees: “The sophistication of the technology calls for new types of law.”
In the past, as new forms of information and disinformation have arisen, society has developed antibodies to deal with them: few people would be fooled by first world war propaganda now. But, says Wachter, the world is changing so fast that we may not be able to develop those antibodies this time around – and even if we do, it could take years, and we have a real problem to sort out right now. “Maybe in 10 years’ time we’ll look back at this stuff and wonder how anyone took it seriously, but we’re not there now.”
• The AI Does Not Hate You by Tom Chivers is published by Weidenfeld & Nicolson (£16.99). To order a copy go to guardianbookshop.com. Free UK p&p on all online orders over £15
What’s New in the create deepfake videos Archives?
Screen Shot
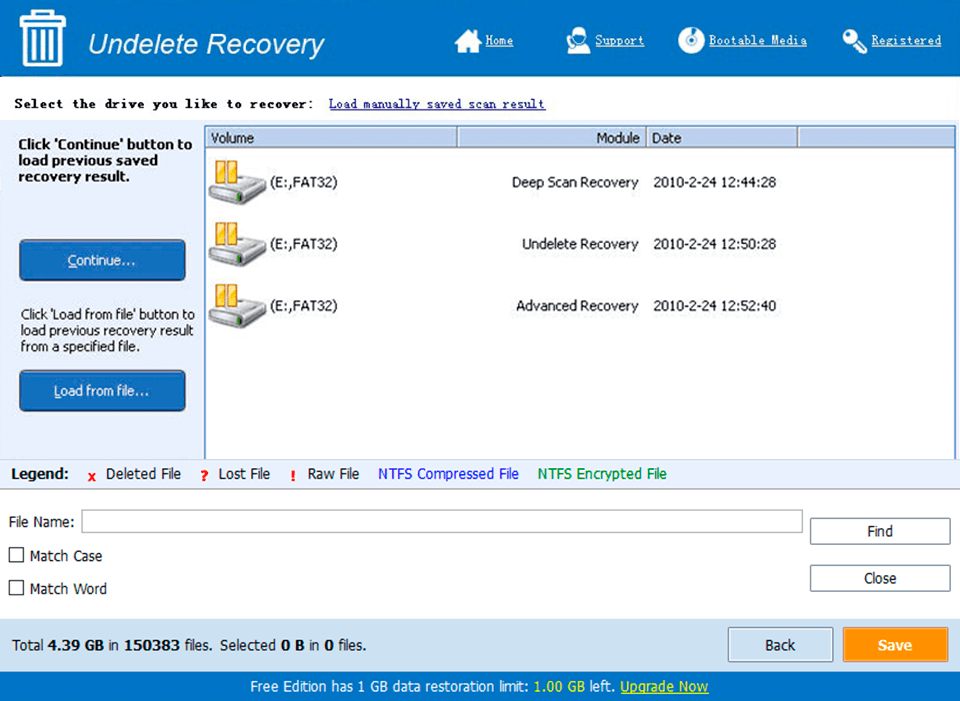
System Requirements for Create deepfake videos Archives
- First, download the Create deepfake videos Archives
-
You can download its setup from given links: